Contextualization in IT - Part 3
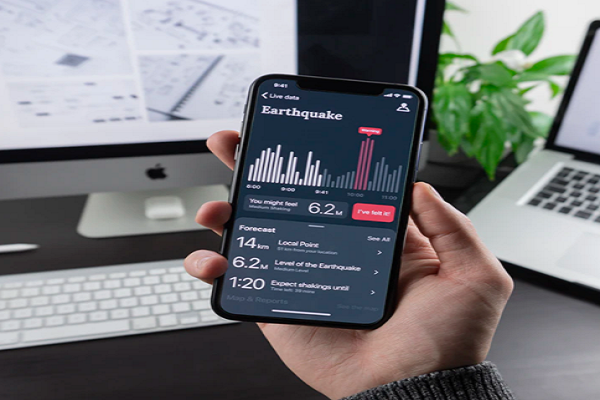
Contextualization in IT Part 3 — How You Could Derive Maximum Value from Enterprise Data
Contextualization is a hot area of investment, with the context-aware computing market expected to reach $158 billion in less than five years. In this series of blogs, I look at the definition of context for modern enterprises, key trends in this space, and strides taken by industry giants like IBM, Google, Amazon, and Oracle.
The third and final part offers an executable roadmap for you to get started with contextualization in IT.
As the number and variety of data sources continue to increase, there is a question of how to generate measurable value from this data. What good is oil, without the pipelines to deliver it or the refineries to convert it into monetizable assets?
Similarly, data is the new oil, and contextualization is the model that will make it value-generating for enterprises at scale. Contextualization leverages three technology components: first is the data source(s) to bring in real-time information. Next, we have the analytics layer that converts it into in-the-moment insights. Finally, we have the visualization layer where the insights are presented in a human-readable format that points the way forward.
I have already discussed these fundamentals in Part 1 if you want to go back and take a look, but today we are exploring a framework to help deploy contextualization at your enterprise.

A 4-Step Contextualization Framework
Before you begin, it is vital to first define the business problem you are trying to address. Unlike large-scale digital transformation, contextualization is almost always a use case-driven initiative. For example, World Wrestling Entertainment (WEE) wanted to generate content that tapped into the exact sentiment of its fans in near real-time. Volkswagen was struggling with fluctuating demand and needed ad-hoc scalability for its digital workplace. Contextualization proved to be an effective answer to these problems — you can read about the solutions in detail in Part 2 of this series.

Once the business problem is in place you can go ahead with data source identification. This could be market intelligence, customer conversations, employee performance metrics, application traffic, network status, or anything else. This is followed by the deployment of the four-step framework:
1. COLLECT — The first step is to gather data from the required sources; you might need additional competencies for unstructured data (big data) if they aren’t already available. For example, optical character recognition (OCR) can help extract data from emails.
2. CONNECT — Data sources must then be connected to surrounding systems to help arrive at a holistic understanding of the context. For instance, cybersecurity data might reveal application traffic anomalies that suggest a threat. It is only when you connect this data to user behaviour information that you can understand which anomalies are permissible as per user behaviour/personas, and which ones need further information.
3. REASON — At this step that you derive the desired insights from data, factoring in the context. Insights can be fed into an automation engine to take decisions and execute them. You can also access them via business dashboards that make contextual insights available to strategic stakeholders. The competencies of your reasoning systems will determine insight accuracy (along with data source coverage).
4. ADAPT — Finally, you need to adapt enterprise operations in line with the insights generated, taking care to update the data sets at regular intervals and refresh your processes accordingly. Over time, this will drive an agile, intuitive enterprise taking contextually relevant decisions at every step.
The Power of Context in Action
The most popular application of context is probably in sales and marketing — thanks to the vast repository of customer data that’s now available via public and third-party sources. But there are other applications as well. Octo Telematics was able to collect IoT data from connected fleets and connect it with traffic and weather data to create a complete context. This helped its insurance customers to assess risk in the automotive segment and verify claims, increasing business for Octo Telematics by 2X times.
Success stories like this are becoming more common as data science becomes democratized. Tools like Augify couple data science with the cloud to create Data Science as a Service platform, bringing the power of context to businesses of every size.
This is an exciting domain, poised for incredible growth in the next half-decade. By taking stock of their data competencies and aligning them with business requirements, enterprises will be able to generate value at every critical touchpoint. Do you agree? Let me know in the comments!